🤖 ChatGPT: more overhyped than robotaxis?
The uncanny valley between GPT-3, autonomous vehicles and the future
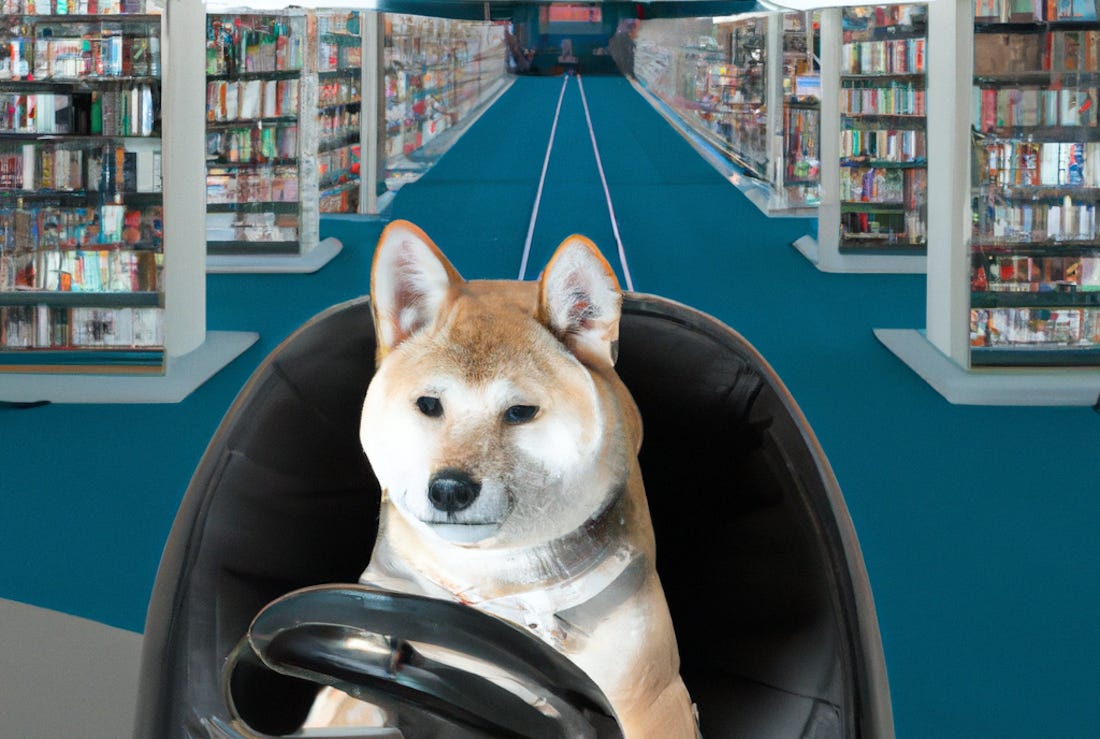
You’re reading the RedBlue Newsletter, which offers deep takes on the intersections between mobility and technology. You can subscribe here:
ChatGPT is uncannily good. It has replaced crypto, now relegated to TMZ, as the hot-topic in tech. ChatGPT has generated stratospheric levels of excitement (and apprehension) with a belief that these systems are widely generalizable.
Autonomous vehicles were once also massively hyped, but the bubble of excitement has deflated. Many are skeptical that autonomous vehicles can scale in the near term to serve many users in many cities.
The contrast in the current sentiment towards these two technologies couldn’t be more striking.
These are two axes of comparison that are helpful in considering how each technology will scale and what the consequences will be.
What is the safety threshold for each technology to permit wide scale deployment?
How complicated is the problem that’s being solved?
Safety threshold
The safety threshold for deploying autonomous vehicles is understandably high. Moving vehicles through our cities and suburbs is dangerous. They have the potential to kill people. Indeed they tragically already have. Autonomous systems need to be extremely reliable to scale.
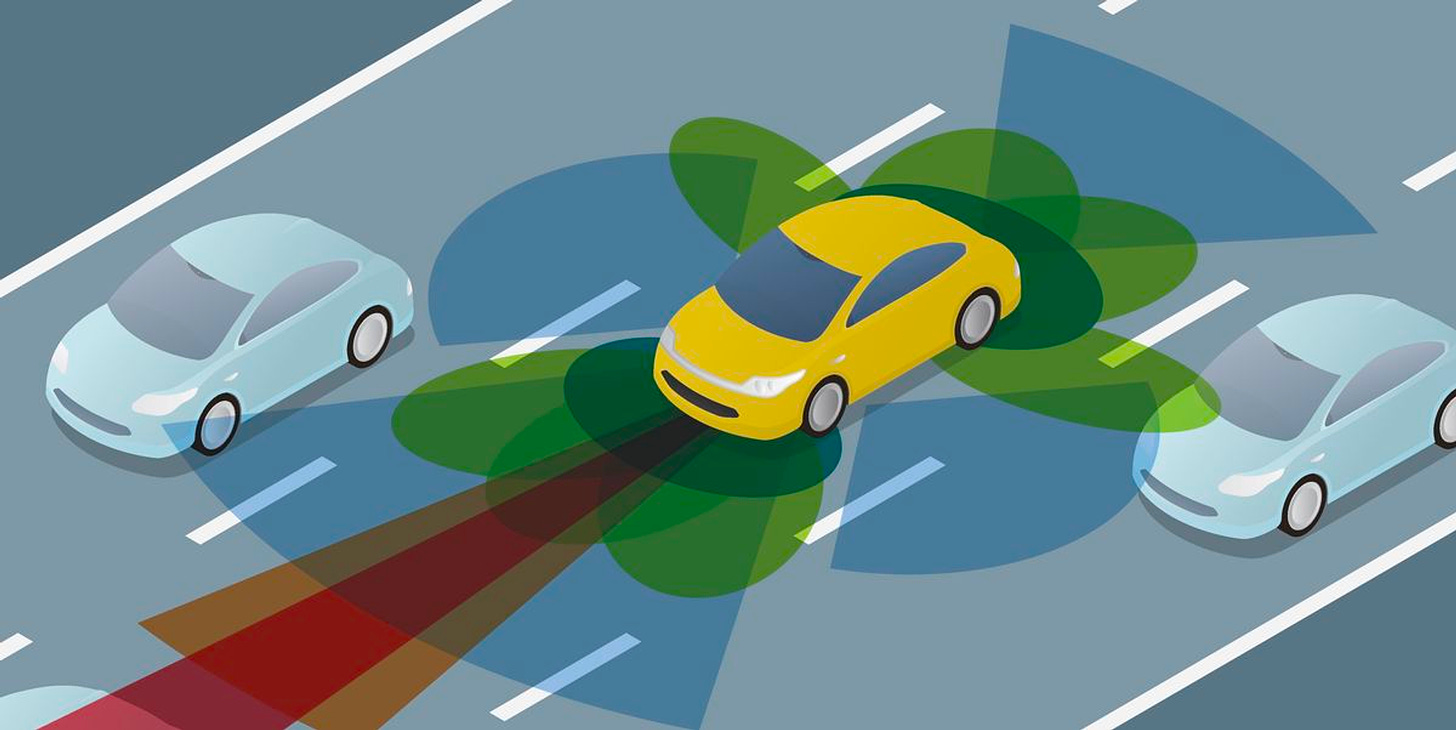
In contrast, GPT seems to have a relatively low bar for deployment: it’s a system that generates text on a screen rather than actuating a vehicle in the physical world. We’ve been doing this kind of thing for a while and it has mostly been harmlessly inept (hey Siri!).
But though the danger appears innocuous, it might be significant. In contrast to autonomous vehicles, where the danger comes from the vehicle itself, the immediate danger with GPT comes from us. It’s really hard to predict how this could play out - as it was hard to predict how social media might lead to a Trump presidency. But it seems that it might be bad in a much more wide reaching way than car crashes are. For instance, truth murder like QAnon or Russian propaganda - which it turns out societies are quite susceptible to - could be significantly amplified if it gets industrialized with neural nets. Longer term, there are reasons to worry about power centralizing around companies like OpenAI, transparency about how these systems work and inbuilt biases in their training data, and alignment between our goals and the outputs of AI.
So we have a high bar with AV safety and a low bar that maybe should be higher with GPT. It seems likely this bar will be raised as unexpected issues start to arise.
Complexity
At first glance, driving might seem cognitively simpler than writing sonnets in Shakespearian verse. There are four directions to direct a car: forwards, backwards, left and right. In contrast, GPT outputs any word and sometimes makes up new ones. And we have had autonomous vehicles that can drive convincingly well for a lot longer than a chat interface that we can convincingly converse with.
However the task of making cars drive themselves has proven devilishly difficult - much harder than anticipated. There is a very long tail of unexpected edge cases (grannies in wheelchairs chasing ducks, people dancing on your car) that are hard to train for or appropriately respond to. Driving also requires understanding the state of mind of other road users. And a lot less of driving—the “training data”—has been digitally recorded than our writing has.
Perhaps the shock of GPT is not so much how complex it is as revealing how unexpectedly simple we are.
On the other hand, even though language appears to “represent a kind of pinnacle of complexity”, as Stephen Wolfram notes in a detailed exploration of GPT, it might actually be simpler than we realize. At least on the surface, ChatGPT seems to do a pretty good job.
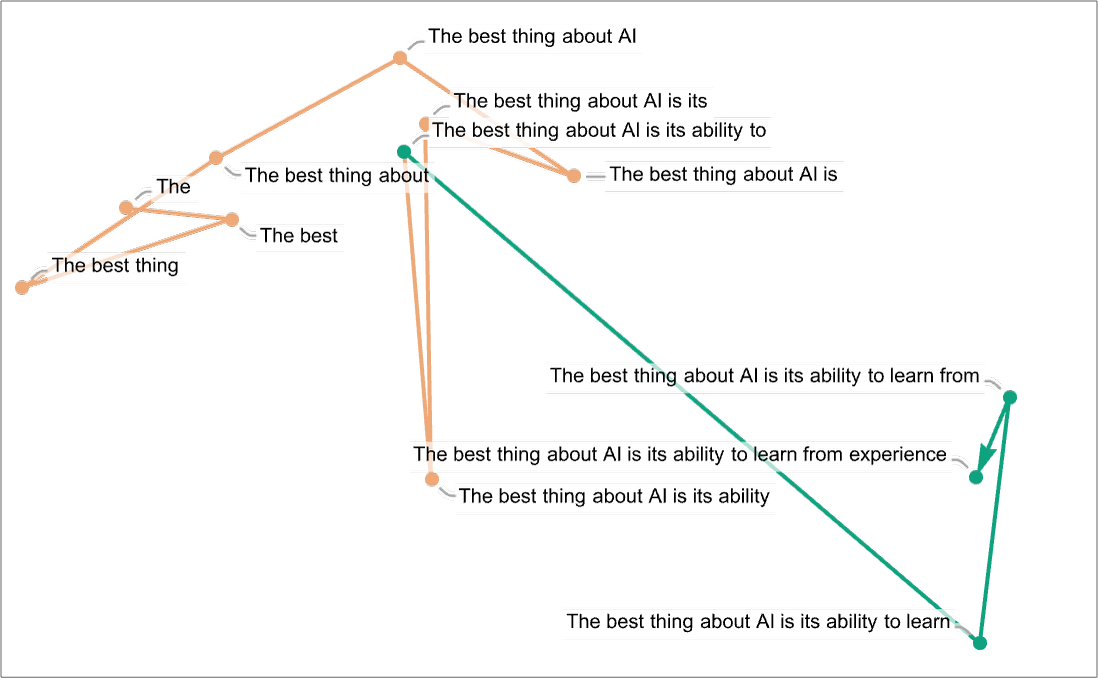
One way to understand the difference between the two problems: AVs have to respond to the unexpected complexities of the real world; GPT only has to map a semantic system we use to model the world. That map covers a lot more things (human knowledge) but it’s been a lot better mapped out than roads in all their dynamic complexity. Language is always a reduction and the way we use it has relatively intuitive internal rules. Perhaps the shock of GPT is not so much how complex it is as revealing how unexpectedly simple we are.
So ChatGPT may be addressing a less hard problem than it would appear - but there are still many ways in which the system is making major errors. And driving is harder than expected - but still it seems like a constrained problem. We at least seem to be not far from having things that work a lot of the time in the case of Waymo and Cruise, at least when coupled with training wheels like remote control.
So autonomous vehicles have to be super safe and it’s a pretty hard problem to solve. But the fact that autonomous vehicles have proven so hard to commercialize suggests that there may be significant challenges as GPT use cases extend into more complex territory or when errors with bad consequences prove hard to quickly remedy.
Path forward
AI is to hype what igloos are to snow. Back in 2015, Sam Altman and Elon Musk discussed autonomous vehicles, claiming that they’d be here “much faster than people think”. Sam suggested they’d be here in 3-4 years. Elon put it closer to 2-3 years. According to most carmakers, we should have had autonomous vehicles roaming our cities two years ago.
It’s worth keeping this hype cycle in mind when thinking about how ChatGPT will evolve. There are three things that might happen next:
GPT develops and improves quickly, while AVs languish
They both develop quickly
They both slow down
Applying neural nets is alchemy: we don’t know why things that work well do so. There’s a lot of tweaking to get better outcomes. Sometimes we have unexpected rapid improvements. But when things go wrong, it’s hard to know why because the system is a black box of billions of numerical weights.
Original approaches to AVs split the driving task into perception, planning and control, but end-to-end approaches are increasingly being applied. GPT uses new things like transformers (the T in GPT). If GPT has made fundamental breakthroughs, these kinds of learnings should percolate across to AVs. So if GPT is on a rapid improvement trajectory, it seems likely that AVs shouldn’t be far behind.
But the performance step up embodied in ChatGPT might be a mirage, especially when imagining a pathway to greater sophistication and especially to AGI. Bing Chat was trained on a larger model than ChatGPT, but its performance seems worse. It’s worth remembering that although ChatGPT appears smart, it is fundamentally dumb: its engine is simply a model that predicts the best word to add next in a text output. It’s not that the improvement pathway will be linear.
A rich history of sci-fi has has seeded many expectations and fears. And it’s clear that these technologies have the potential to create massive change and generate massive value. When it works, it’s hard to distinguish from magic. When it doesn’t, it’s also hard to understand why or how to fix it. A lesson from autonomous vehicles is that we shouldn’t let sentiments get ahead of us: appearances can be uncanny.